Advanced solutions for information modeling and data integration
In our highly connected world, governments and corporations with complex data integration challenges are looking for advanced solutions for sharing and integrating data between departments, agencies, suppliers and customers.
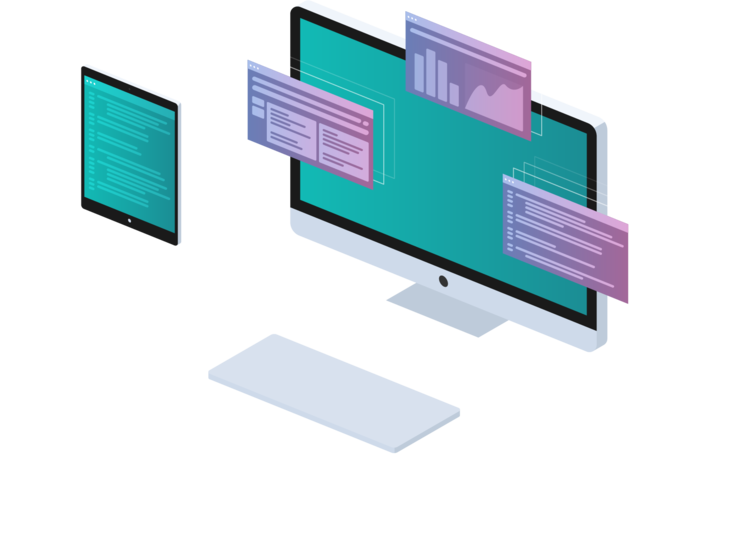
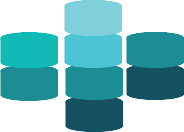
Today most data is locked up in data silos, making it hard to integrate, exchange and share:
- Modelled and encoded differently,
- Exposed to data quality issues,
- Data matching without entity ids is imprecise and error prone
- ... but data matching accuracy is rarely measured.
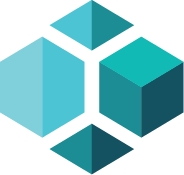
Factil provides advanced solutions for:
- Modeling information in a business-oriented language,
- Machine learning and probabilistic data matching,
- Statistically rigorous data matching evaluation,
- Building 360° view of customer/citizen
that can be readily integrated with existing Master Data Management and data warehouse solutions.
Advise, analyse, design, implement and support
We help organisations with complex data integration challenges to model their business information and deliver leading-edge data sharing and integration solutions.
Data matching is at the heart of any data sharing or integration solution
Data matching without entity ids is inherently imprecise
- Key business entities such as Person and Address do not have global unique entity ids.
- Data matching is based on attributes and related data.
- Exposed to data quality issues,
- Each data domain has specific typographical, phonetic, cultural and technical challenges.
Use advanced algorithms for record matching
- Logistic Regression is a machine learning technique to calculate the ideal feature weights against a reference training set.
- Probabilistic Matching uses on statistical techniques for assessing the probability that any pair of records represent the same entity.
Data matching is error prone, but rarely measured
Two kinds of errors:
- False positives: Two records that do not correspond to the same entity, but the system claims they are a match.
- False negatives: Two records correspond to the same entity, but the system claims they are not a match.
Apply statistically rigourous data matching evaluation
- Matching accuracy is measured in terms of precision and recall
- Accuracy is calculated by comparing the results returned by the matching algorithm to reference evaluaiton set that has been previously labelled by a human expert.
Build 360° view of customer or citizen
Currently building 360° view of customer or citizen is typically based on using traditional Master Data Management (MDM) solutions.
But traditional MDM solutions rarely measure matching accuracy and are prone to false negative errors.
But help is at hand by integrating Factil's data matching and matching evaluation technologies with existing MDM solutions.
Augment current Master Data Management solutions
- Find a higher proportion of hard-to-find record matches
- Automate clerical review decisions that would otherwise be done manually
- Formally measure entity resolution accuracy